This all started when a friend of mine linked me to this Quora article she found interesting: How does the tinder algorithm work?
- 5 Ways the Tinder Algorithm Can Work for You?
- Tinder's Algorithm / How the "ELO Score" is calculated & What you can do to improve yours?
- The 3 Main Elements.
Alex Mark then theorised the following from his own logic and review of the app: These first cards will also be significantly more attractive than the general population of tinder users as a whole. If you have a low number of matches, tinder will continue to show you non-matches, but much less attractive than the first group. Alex Mark further delves into how Tinder will show you more attractive profiles if you yourself are more attractive, and how being more active on the app will let you appear more often to other people, thus increasing your chances of matching.
Technologies are not merely the outcomes of human agency, they affect it as well. In the context of algorithmically-driven online platforms, two categories of human actors are considered: Both categories of human actors actively interface with algorithmic systems whose development is increasingly outsourced to machine learning algorithms.
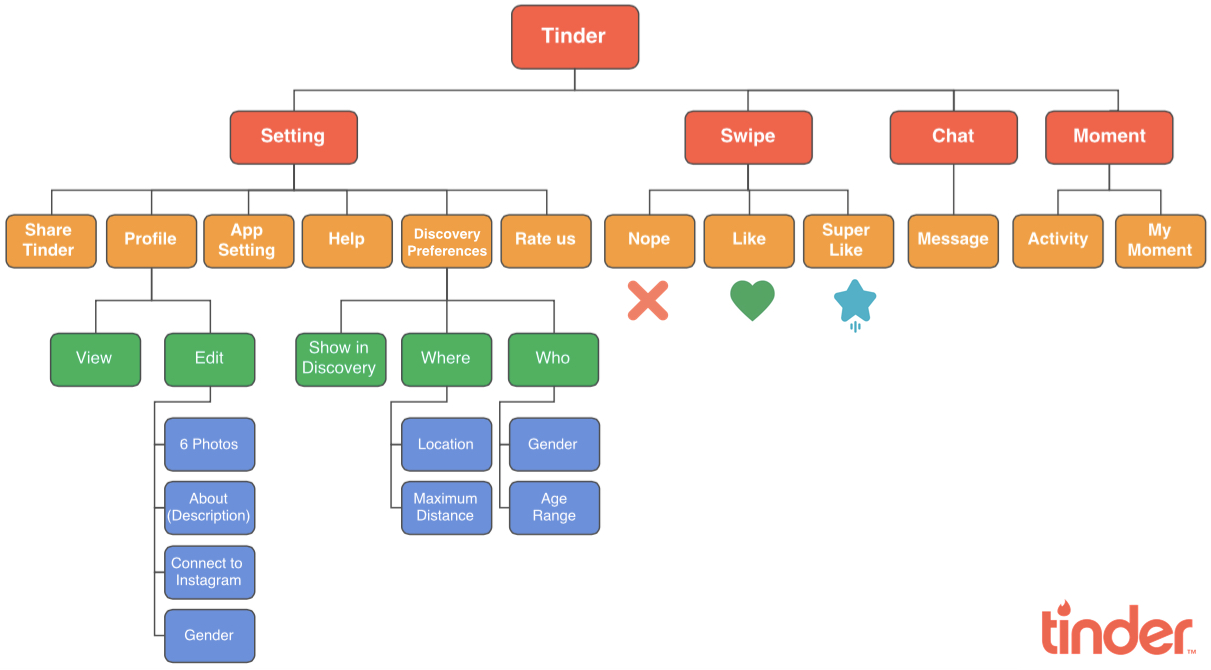
More specifically, most platform owners and developers plan out a concept for a service and, as its earliest users, develop and refine its initial platform mechanics. In the first phase, the efforts are directed towards carefully constructing an attractive discourse that creates buzz, seeking out a growing user base Gillespie, However, while progressing in the diffusion cycle, the quest for a viable business model prevails, especially when investors require a return.
After all, a large set of users actively fuels further business development which translates into economic value.
How Tinder Works, And Why You're Getting More Matches Than Before
Revenue is generated either directly through paying users, or indirectly e. This sequence of goals forms the internal-structural backdrop against which platform owners and developers exercise agency. Algorithms, and machine learning algorithms in particular, inform or even partly account for these choices.
Algorithms with independent learning capabilities are used to enhance a service by offering elaborate means for real-time meta data-analysis. They are able to assist in selecting interface features, default settings, protocols, and continually tweaking platform algorithms. For instance, platform owners set out a desired outcome e. The process of machine learning is metaphorically equivalent to a farmer who sows crops, caters for the resources and conditions for them to grow, while eventually harvesting and selling the yield Domingos, Accordingly, to feed these mechanisms, there is a need for an incessant stream of refined user data.
In that sense, platform development is referred to as a virtuous circle of big data Harrison, This means that absolute control over their technological structures is obscured: Mackenzie suggests that the uptake of machine learning characterizes a shift from absolute control over data to the tendency to outsource control to data. Still, algorithms remain indebted to platform owners and developers as they set out the boundaries and the corporate strategy in which these technologies function. Platform users exercise agency within the boundaries that a platform provides: Still, users are not powerless in this relation, albeit to differing degrees, depending on their nature of using the platform i.
First, as algorithms run on data, users are the key resource for them to learn and improve. Atypical user behavior, such as trying to play or trick algorithms, might provoke outcomes users specifically desire.
The factors that affect your score/ranking/visibility:
For instance, by inconsistently liking objects on Facebook, users can try to confuse the algorithm in learning about consumer preference, which distorts individualized advertising Bucher, Such behavior has the potential to disrupt technological structures implicitly, rather than sustaining them as they are. Moreover, some platforms explicitly allow user control and give feedback on a personalized information stream e.
Even more, beyond platform protocols, the widespread uptake of specific user practices can entice the development of new formal features e. Within the academic literature, the relationship between platforms owners and their algorithms is covered by critical studies into the political economy of online platforms and platform studies. The former is engaged with uncovering mechanisms of user commodification and digital labor e. Platform studies, on the other hand, widely focus on platform evolutions in technological interfaces, default settings, protocols, algorithms, and metadata, as well as the discourses that characterize these platforms e.
Tinder’s Algorithm / How the “ELO Score” is calculated & What you can do to improve yours
Most notably, van Dijck disassembles platforms as techno-cultural constructs and socio-economic structures. She considers platforms as technological infrastructures with specific rules and resources that, together with their users, produce social outcomes by drawing upon the contents the users provide. This process is rooted within an economic logic, in which ownership, governance, and business model development compose the context in which the aforementioned process takes place.
These perspectives provide the opportunity to take on the viewpoint of platform owners and developers, allowing to understand their internal structures and consequently their actions. This knowledge is especially valuable for media effects research, which traditionally focuses on users, but currently falls short in incorporating algorithmic governance into its conceptual and empirical models. Due to the influence of algorithms, exposure on algorithmically-governed platforms is highly individualized, hardly transparent and perhaps even involuntary. It is a function of user action, but not its direct result.
This makes it hard to infer whether and to what extent exposure is molded by platform algorithms, thus obscuring the effects that follow from it. It is difficult to assess which factors provoke this, and how they can be resisted or turned around. Conceptually, we argue that media exposure on online platforms is an effect produced by both user action and algorithmic processing, which in turn likely provokes other effects e.
What this algorithmic processing involves is largely unknown as platforms rarely inform the public. However, the technological and economic logics that pressure online platforms could help us to generate testable hypotheses on what algorithms possibly do. Systematic research on the dynamics of algorithmically-governed online platforms is challenging because of the proprietary, closed off nature of such environments.
Even if such unrestricted platform data collection were possible, it still lacks valuable information as it is generally limited to behavioral data and hardly informs on the social and psychological effects that platform exposure brings about in its users. We need a method that overcomes these problems in grasping user actions, exposure and effects on online platforms.
Experience sampling method ESM is a feasible candidate to alleviate at least some of these methodological limitations. A mobile device prompts a panel of participants to repeatedly fill out a compact questionnaire form on the most recent relevant experience. In this case, that would ideally be immediately after a platform is used. The ESM format allows for a limited set of questions on: In this case, the ESM questionnaire form should involve the most important algorithmic input variables. It is equally important to capture the algorithmically-governed exposure i.
This allows for general inferences concerning the underlying mechanism, despite the inability to directly examine this black box. These inferences are ideally based on conceptually sound hypotheses. This is where insights from platform studies and critical studies on the political economy of online media come into play Figure 1 , top half.
It should be possible to construct informed assumptions on the mechanics of algorithms by considering the economic and technological logics that pressure platform owners and developers. Still, this format could suffice in at least grasping its general dynamics. Moreover, in contrast to digital methods research, major advantages of this approach are the independence from platform APIs to collect data and the opportunity to move beyond behavioral data by delving into otherwise inaccessible social and psychological consequences through self-report measures.
The issue of bias in self-report data persists, albeit to a lesser extent due to the reduced time interval between exposure and data collection Hektner et al. As a proof of concept, four hypotheses are developed for the case of Tinder Figure 1 , bottom half. We choose Tinder because the commercial platform is currently restricted to mobile devices, and because it draws upon a limited number of explicit input and output variables. Also, the mobile app invites considerable speculation on how it operates Duguay, Despite its efforts to construct a discourse of a fresh, bottom-up success story Summers, , Tinder was internally conceived within an incubator program, nested in InterActiveCorp IAC, This large media company owns a broad repertoire of global online brands, such as Vimeo and HomeAdvisor.
It includes the Match Group IPO in that holds an impressive global portfolio of over 45 online dating platforms, based on various architectures and business models that target a wide range of consumer types e. As a global brand, Tinder presents itself towards the supposedly unserved audience of emerging adults. It aims to be a playful means to instigate new contacts, directed to lower the practical and social barriers that characterize traditional dating platforms MatchGroup, Since its inception, Tinder has been part of a well-thought through marketing strategy.
Its uptake was promoted through local events, such as Tinder-themed parties Bosker, This has been a key objective right from the beginning: Users log on with their Facebook account, and quickly set up a profile that is predominantly made up by pictures. Moreover, they can indicate their sexual preferences, and determine the age range and the location radius in which they want to assess profiles. This assessment process is swift and playful: The swiping process on Tinder remains anonymous until both users right swipe like each other, which means they match.
Only after matching, are users allowed to initiate further contact through an instant messaging module. This implies that the design of Tinder is strongly focused on a dynamic of mutual attraction and consent MacKee, Despite its simple appearance, there is more to Tinder than meets the eye. The platform draws upon algorithmic filtering, which curates whom gets to like whom, and when this happens. Although Tinder rarely communicates about its underlying algorithm, it does admit that each user has an individual attractiveness score, which is opaquely computed on the basis of popularity and user behavior indices Kosoff, However, Tinder has been experimenting with advertising, while gradually implementing paid services.
Tinder is now a freemium app, offering basic functionality to non-paying users, while premium features are available through subscription i. Tinder Plus is a monthly, bi-annual or annual subscription to a series of features, including unlimited likes, an increased number of Super Likes, the possibility to undo left swipes, flexible location settings and Tinder Boost. Super Likes are a scarce resource that allow for indicating special interest in a profile. This feature is advertised as increasing the likelihood of getting matches Tinder, In essence, Tinder entices users by letting them swipe through interesting profiles.
The key is to tease users by offering a wide range of fairly realistic opportunities.
- Explained: How Tinder Picks Works [And How To Get It]?
- Introduction.
- dating site for working out?
Based on Zhang , we assume Tinder carefully doses matches, meaning that its governing algorithm monitors activity and intervenes in its outcomes to keep the user experience in check. Especially for the free service, the key is to keep users sufficiently satisfied so they do not abandon the service too quickly, but not too satisfied so they would be inclined to convert to paying services.